JonBenet Ramsey trace evidence, touch DNA and Bayesian inference
The Unsolved Murder of JonBenet Ramsey :: The Unsolved Murder of JonBenet Ramsey-BLOGS :: Redpill's Blog
Page 1 of 1
JonBenet Ramsey trace evidence, touch DNA and Bayesian inference

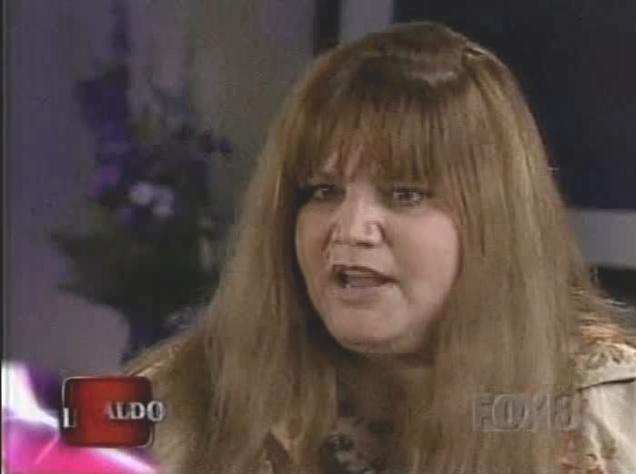
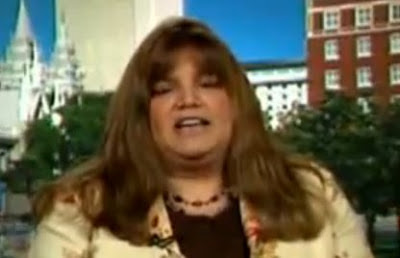
this is what she claims
http://www.websleuths.com/forums/showthread.php?76520-Patsy-Ramsey/page92
tricia griffith wrote:
Anti-K, this whole forum has example after example after example that an intruder did not commit this crime.
No one can show one scintilla of evidence of an intruder.
As owner, I do my best to stay out of actual discussions about a crime.
The JBR case is the one expection.
Websleuths is a leader in true crime information as well as discussion. People come here to get information. It is imperative we deal with the facts. Not fantasy.
All I ask for are facts and a logical connecting of the dots. Logic and facts.
When I get time I will be going through the forum to make sure the JonBenet Ramsey forum is being held up to the high standards just like all our other forums on Websleuths.
The days of allowing anyone to post anything because it's part of their "theory" are gone. Facts and logic. Very simple.
this is her qualifications
Host Tricia Griffith is a veteran radio disc jockey and owner of Websleuths.com and owner of Forums for Justice.org.
in other words she has ZERO qualifications in forensic science. she has no training in forensic fiber, trace evidence, DNA yet she claims
tricia griffith wrote:
Anti-K, this whole forum has example after example after example that an intruder did not commit this crime.
No one can show one scintilla of evidence of an intruder.
let's quickly review her qualification again
Host Tricia Griffith is a veteran radio disc jockey and owner of Websleuths.com and owner of Forums for Justice.org.
when it comes to RDI vs IDI, one thing to keep in mind is anyone can hear from a friend that they saw a documentary that proves Burke hit Jonbenet over the head with a flashlight after Jonbenet stole Burke's pineapple, and the family covered it up, with the ransom note as part of the coverup.
even high school dropouts, and i suspect many RDI are dropouts, or never attended college and majored in hard physical sciene, has done so.
what you will never see any RDI do is catalogue the trace evidence, us a scientifically approved methodology and scientific reasoning on the trace forensic evidence, in deciding between RDI vs IDI. instead RDI just say, the parents did it, no i think burke did it, and they wrote the ransom note to cover it up.
they never discuss the unsourced trace evidence found on Jonbenet, in totallity, and apply scientific reasoning as to how best to explain how that trace evidence, and other evidence, got there.
one way to evaluate this is using Bayesian inference
the following is all from wikipedia
In probability theory and statistics, Bayes’ theorem (alternatively Bayes’ law or Bayes' rule) describes the probability of an event, based on prior knowledge of conditions that might be related to the event. For example, if cancer is related to age, then, using Bayes’ theorem, a person’s age can be used to more accurately assess the probability that they have cancer, compared to the assessment of the probability of cancer made without knowledge of the person's age.
One of the many applications of Bayes’ theorem is Bayesian inference, a particular approach to statistical inference. When applied, the probabilities involved in Bayes’ theorem may have different probability interpretations. With the Bayesian probability interpretation the theorem expresses how a subjective degree of belief should rationally change to account for availability of related evidence. Bayesian inference is fundamental to Bayesian statistics.
Bayes’ theorem is named after Rev. Thomas Bayes (/ˈbeɪz/; 1701–1761), who first provided an equation that allows new evidence to update beliefs. It was further developed by Pierre-Simon Laplace, who first published the modern formulation in his 1812 “Théorie analytique des probabilités.” Sir Harold Jeffreys put Bayes’ algorithm and Laplace's formulation on an axiomatic basis. Jeffreys wrote that Bayes’ theorem “is to the theory of probability what the Pythagorean theorem is to geometry.”[1]
Examples[edit]
Drug testing[edit]
Tree diagram illustrating drug testing example. U, Ū, "+" and "−" are the events representing user, non-user, positive result and negative result. Percentages in parentheses are calculated.
Suppose a drug test is 99% sensitive and 99% specific. That is, the test will produce 99% true positive results for drug users and 99% true negative results for non-drug users. Suppose that 0.5% of people are users of the drug. What is the probability that a randomly selected individual with a positive test is a user?
Despite the apparent accuracy of the test, if an individual tests positive, it is more likely that they do not use the drug than that they do. This surprising result arises because the number of non-users is very large compared to the number of users; thus the number of false positives outweighs the number of true positives. To use concrete numbers, if 1000 individuals are tested, there are expected to be 995 non-users and 5 users. From the 995 non-users, 0.01 × 995 ≃ 10 false positives are expected. From the 5 users, 0.99 × 5 ≈ 5 true positives are expected. Out of 15 positive results, only 5, about 33%, are genuine. This illustrates the importance of base rates, and how the formation of policy can be egregiously misguided if base rates are neglected.[15]
The importance of specificity in this example can be seen by calculating that even if sensitivity is raised to 100% and specificity remains at 99% then the probability of the person being a drug user only rises from 33.2% to 33.4%, but if the sensitivity is held at 99% and the specificity is increased to 99.5% then probability of the person being a drug user rises to about 49.9%.
A more complicated example[edit]
The entire output of a factory is produced on three machines. The three machines account for different amounts of the factory output, namely 20%, 30%, and 50%. The fraction of defective items produced is this: for the first machine, 5%; for the second machine, 3%; for the third machine, 1%. If an item is chosen at random from the total output and is found to be defective, what is the probability that it was produced by the third machine?
A solution is as follows. Let Ai denote the event that a randomly chosen item was made by the ith machine (for i = 1,2,3). Let B denote the event that a randomly chosen item is defective. Then, we are given the following information:
P(A1) = 0.2, P(A2) = 0.3, P(A3) = 0.5.
If the item was made by the first machine, then the probability that it is defective is 0.05; that is, P(B | A1) = 0.05. Overall, we have
P(B | A1) = 0.05, P(B | A2) = 0.03, P(B | A3) = 0.01.
To answer the original question, we first find P(B). That can be done in the following way:
P(B) = Σi P(B | Ai) P(Ai) = (0.05)(0.2) + (0.03)(0.3) + (0.01)(0.5) = 0.024.
Hence 2.4% of the total output of the factory is defective.
We are given that B has occurred, and we want to calculate the conditional probability of A3. By Bayes' theorem,
P(A3 | B) = P(B | A3) P(A3)/P(B) = (0.01)(0.50)/(0.024) = 5/24.
Given that the item is defective, the probability that it was made by the third machine is only 5/24. Although machine 3 produces half of the total output, it produces a much smaller fraction of the defective items. Hence the knowledge that the item selected was defective enables us to replace the prior probability P(A3) = 1/2 by the smaller posterior probability P(A3 | B) = 5/24.
Once again, the answer can be reached without recourse to the formula by applying the conditions to any hypothetical number of cases. For example, if 100,000 items are produced by the factory, 20,000 will be produced by Machine A, 30,000 by Machine B, and 50,000 by Machine C. Machine A will produce 1000 defective items, Machine B 900, and Machine C 500. Of the total 2400 defective items, only 500, or 5/24 were produced by Machine C.
Example[edit]
Tree diagram illustrating frequentist example. R, C, P and P bar are the events representing rare, common, pattern and no pattern. Percentages in parentheses are calculated. Note that three independent values are given, so it is possible to calculate the inverse tree (see figure above).
An entomologist spots what might be a rare subspecies of beetle, due to the pattern on its back. In the rare subspecies, 98% have the pattern, or P(Pattern | Rare) = 98%. In the common subspecies, 5% have the pattern. The rare subspecies accounts for only 0.1% of the population. How likely is the beetle having the pattern to be rare, or what is P(Rare | Pattern)?
From the extended form of Bayes’ theorem (since any beetle can be only rare or common),
Bayesian inference is a method of statistical inference in which Bayes' theorem is used to update the probability for a hypothesis as more evidence or information becomes available. Bayesian inference is an important technique in statistics, and especially in mathematical statistics. Bayesian updating is particularly important in the dynamic analysis of a sequence of data. Bayesian inference has found application in a wide range of activities, including science, engineering, philosophy, medicine, sport, and law. In the philosophy of decision theory, Bayesian inference is closely related to subjective probability, often called "Bayesian probability".
Examples[edit]
Probability of a hypothesis[edit]
Suppose there are two full bowls of cookies. Bowl #1 has 10 chocolate chip and 30 plain cookies, while bowl #2 has 20 of each. Our friend Fred picks a bowl at random, and then picks a cookie at random. We may assume there is no reason to believe Fred treats one bowl differently from another, likewise for the cookies. The cookie turns out to be a plain one. How probable is it that Fred picked it out of bowl #1?
Intuitively, it seems clear that the answer should be more than a half, since there are more plain cookies in bowl #1. The precise answer is given by Bayes' theorem. Let {\displaystyle H_{1}} H_{1} correspond to bowl #1 ....
Making a prediction[edit]
Example results for archaeology example. This simulation was generated using c=15.2.
An archaeologist is working at a site thought to be from the medieval period, between the 11th century to the 16th century. However, it is uncertain exactly when in this period the site was inhabited. Fragments of pottery are found, some of which are glazed and some of which are decorated. It is expected that if the site were inhabited during the early medieval period, then 1% of the pottery would be glazed and 50% of its area decorated, whereas if it had been inhabited in the late medieval period then 81% would be glazed and 5% of its area decorated. How confident can the archaeologist be in the date of inhabitation as fragments are unearthed?
The degree of belief in the continuous variable
In frequentist statistics and decision theory[edit]
A decision-theoretic justification of the use of Bayesian inference was given by Abraham Wald, who proved that every unique Bayesian procedure is admissible. Conversely, every admissible statistical procedure is either a Bayesian procedure or a limit of Bayesian procedures.[11]
Wald characterized admissible procedures as Bayesian procedures (and limits of Bayesian procedures), making the Bayesian formalism a central technique in such areas of frequentist inference as parameter estimation, hypothesis testing, and computing confidence intervals.[12] For example:
"Under some conditions, all admissible procedures are either Bayes procedures or limits of Bayes procedures (in various senses). These remarkable results, at least in their original form, are due essentially to Wald. They are useful because the property of being Bayes is easier to analyze than admissibility."[11]
"In decision theory, a quite general method for proving admissibility consists in exhibiting a procedure as a unique Bayes solution."[13]
"In the first chapters of this work, prior distributions with finite support and the corresponding Bayes procedures were used to establish some of the main theorems relating to the comparison of experiments. Bayes procedures with respect to more general prior distributions have played a very important role in the development of statistics, including its asymptotic theory." "There are many problems where a glance at posterior distributions, for suitable priors, yields immediately interesting information. Also, this technique can hardly be avoided in sequential analysis."[14]
"A useful fact is that any Bayes decision rule obtained by taking a proper prior over the whole parameter space must be admissible"[15]
"An important area of investigation in the development of admissibility ideas has been that of conventional sampling-theory procedures, and many interesting results have been obtained."[16]
In the courtroom[edit]
Bayesian inference can be used by jurors to coherently accumulate the evidence for and against a defendant, and to see whether, in totality, it meets their personal threshold for 'beyond a reasonable doubt'.[21][22][23] Bayes' theorem is applied successively to all evidence presented, with the posterior from one stage becoming the prior for the next. The benefit of a Bayesian approach is that it gives the juror an unbiased, rational mechanism for combining evidence. It may be appropriate to explain Bayes' theorem to jurors in odds form, as betting odds are more widely understood than probabilities. Alternatively, a logarithmic approach, replacing multiplication with addition, might be easier for a jury to handle.
Adding up evidence.
If the existence of the crime is not in doubt, only the identity of the culprit, it has been suggested that the prior should be uniform over the qualifying population.[24] For example, if 1,000 people could have committed the crime, the prior probability of guilt would be 1/1000.
Gardner-Medwin[25] argues that the criterion on which a verdict in a criminal trial should be based is not the probability of guilt, but rather the probability of the evidence, given that the defendant is innocent (akin to a frequentist p-value). He argues that if the posterior probability of guilt is to be computed by Bayes' theorem, the prior probability of guilt must be known. This will depend on the incidence of the crime, which is an unusual piece of evidence to consider in a criminal trial. Consider the following three propositions:
A The known facts and testimony could have arisen if the defendant is guilty
B The known facts and testimony could have arisen if the defendant is innocent
C The defendant is guilty.
Gardner-Medwin argues that the jury should believe both A and not-B in order to convict. A and not-B implies the truth of C, but the reverse is not true. It is possible that B and C are both true, but in this case he argues that a jury should acquit, even though they know that they will be letting some guilty people go free. .
Other[edit]
The scientific method is sometimes interpreted as an application of Bayesian inference. In this view, Bayes' rule guides (or should guide) the updating of probabilities about hypotheses conditional on new observations or experiments.[27]
now imagine a forensic scientist with expertise in crime scene reconstruction, trace evidence analysis, shoe print, DNA, handwriting and lingustics, all of whom qualify under Daubert
all rigorously trained in Bayesian inference were to decide between to competing explanations for the trace evidence, one explanation is an inside job, and one, an intruder.
how does this Bayesian inference based on UNSOURCED fiber, shoe print, animal hair, handwriting, linguistics of the ransom note, DNA
what is the Bayesian inference of a theory that an intruder enters the Ramsey home, makes contact with Jonbenet, and leaves behind fiber, hair, DNA ligature tape
vs RDI inference
which of the 2 scientific explanations better explains all of the unsourced items and trace evidence?
the answer is clear
on scientific forensic grounds, Jonbenet was murdered by an intruder, which more completely explains all of the UNSOURCED evidence left behind and handwriting and linguistics
of the tens of millions of RDI posters on websleuth topix forumsforjustice reddit justicequest etc., you will never hear any discussion of Bayesian inference on the forensic evidence, bc they are ignorant of something so central in evaluating forensic evidence using science and scientific methology.
they all failed Jonbenet. they are an anti-science lynch mob
forumsforjustice is forumsforjackasses
you've been redpilled.
_________________
If you only knew the POWER of the Daubert side
redpill- Posts : 6201
Join date : 2012-12-08

» RDI is touch DNA in The Unsolved Murder of JonBenet Ramsey
» touch DNA and JonBenet Ramsey questions for RDI same scientific methodology
» touch DNA and JonBenet Ramsey example 2 RDI websleuth forumsforjustice and Locard's exchange principle
» Why touch DNA is scientific forensic evidence of an intruder in JonBenet Ramsey 2
» RDI and touch DNA in other crimes Rosie Tapia 6 & The Unsolved Murder of JonBenet Ramsey
» touch DNA and JonBenet Ramsey questions for RDI same scientific methodology
» touch DNA and JonBenet Ramsey example 2 RDI websleuth forumsforjustice and Locard's exchange principle
» Why touch DNA is scientific forensic evidence of an intruder in JonBenet Ramsey 2
» RDI and touch DNA in other crimes Rosie Tapia 6 & The Unsolved Murder of JonBenet Ramsey
The Unsolved Murder of JonBenet Ramsey :: The Unsolved Murder of JonBenet Ramsey-BLOGS :: Redpill's Blog
Page 1 of 1
Permissions in this forum:
You cannot reply to topics in this forum
|
|